Robots on the rise? AI is nothing new for climate research
Time is short to act on climate change. AI methods have dramatically increased the pace of climate research.
AI models are used to detect small water bodies in Arctic landscapes.
image by Andrew Mullen
“It’s been around a long time, actually,” muses Senior Scientist, Dr. Jennifer Francis. “It’s gotten more sophisticated, sure, and a lot of the applications are new. But the concept of artificial intelligence is not.”
Francis has been working with it for almost two decades, in fact. Although, back when she started working with a research tool called “neural networks,” they were less widely known in climate science and weren’t generally referred to as artificial intelligence.
But recently, AI seems to have come suddenly out of the woodwork, infusing nearly every field of research, analysis, and communication. Climate science is no exception. From mapping thawing Arctic tundra, to tracking atmospheric variation, and even transcribing audio interviews into text for use in this story, AI in varying forms is woven into the framework of how Woodwell Climate creates new knowledge.
AI helps climate scientists track trends and patterns
The umbrella term of artificial intelligence encompasses a diverse set of tools that can be trained to do tasks as diverse as imitating human language (à la ChatGPT), playing chess, categorizing images, solving puzzles, and even restoring damaged ancient texts.
Francis uses AI to study variations in atmospheric conditions, most recently weather whiplash events— when one stable weather pattern suddenly snaps to a very different one (think months-long drought in the west disrupted by torrential rain). Her particular method is called self-organizing maps which, as the name suggests, automatically generates a matrix of maps showing atmospheric data organized so Francis can detect these sudden snapping patterns.
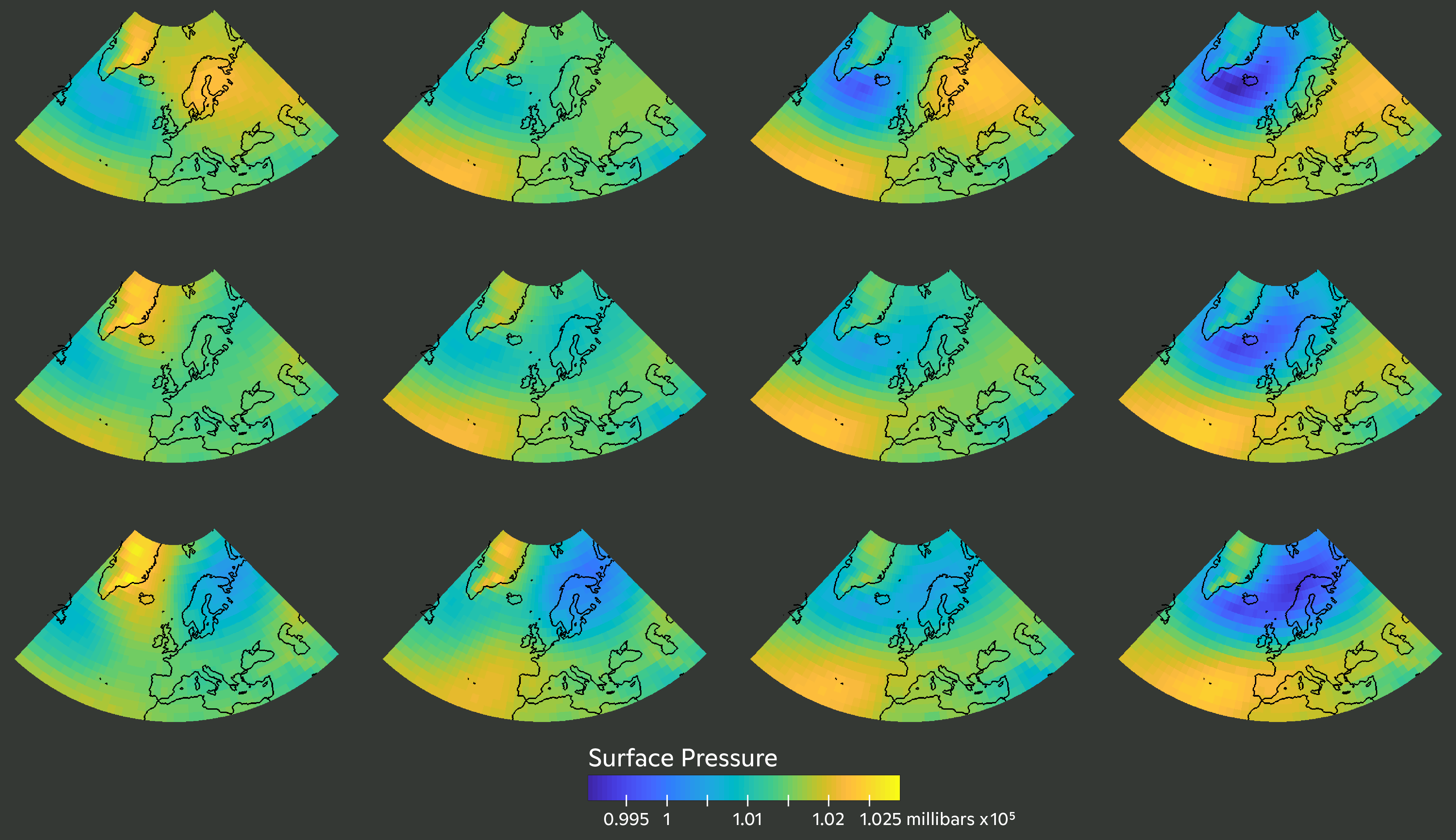
Self organizing maps show major patterns in atmospheric pressure, organized by similarity. A whiplash event is indicated by a sudden change from one pattern to another not immediately touching it.
graphic by Jennifer Francis, Christina Shintani
“This method is perfect for what we’re looking for because it removes the human biases. We can feed it daily maps of, say, what the jetstream looks like, and then the neural network finds characteristic patterns and tells us exactly which days the atmosphere is similar to each pattern. There are no assumptions,” says Francis.
This aptitude for pattern recognition is a core function of many types of neural networks. In the Arctic program, AI is used to churn through thousands of satellite images to detect patterns that indicate specific features in the landscape using a technique originally honed for use in the medical industry to read CT scan images.
Data science specialist, Dr. Yili Yang, uses AI models trained to identify features called retrogressive thaw slumps (RTS) in permafrost-rich regions of the Arctic. Thaw slumps form in response to subsiding permafrost and can be indicators of greater thawing on the landscape, but they are hard to identify in images.
“Finding one RTS is like finding a single building in a city,” Yang says. It’s time consuming, and it really helps if you already know what you’re looking for. Their trained neural network can pick the features out of high-resolution satellite imagery with fairly high accuracy.
Research Assistant Andrew Mullen uses a similar tool to find and map millions of small water bodies across the Arctic. A neural network generated a dataset of these lakes and ponds so that Mullen and other researchers could track seasonal changes in their area.
And there are opportunities to use AI not just for the data creation side of research, but trend analysis as well. Associate Scientist Dr. Anna Liljedahl leads the Permafrost Discovery Gateway project which used neural networks to create a pan-Arctic map of ice wedge polygons—another feature that indicates ice-rich permafrost in the ground below and, if altered over time, could suggest permafrost thaw.
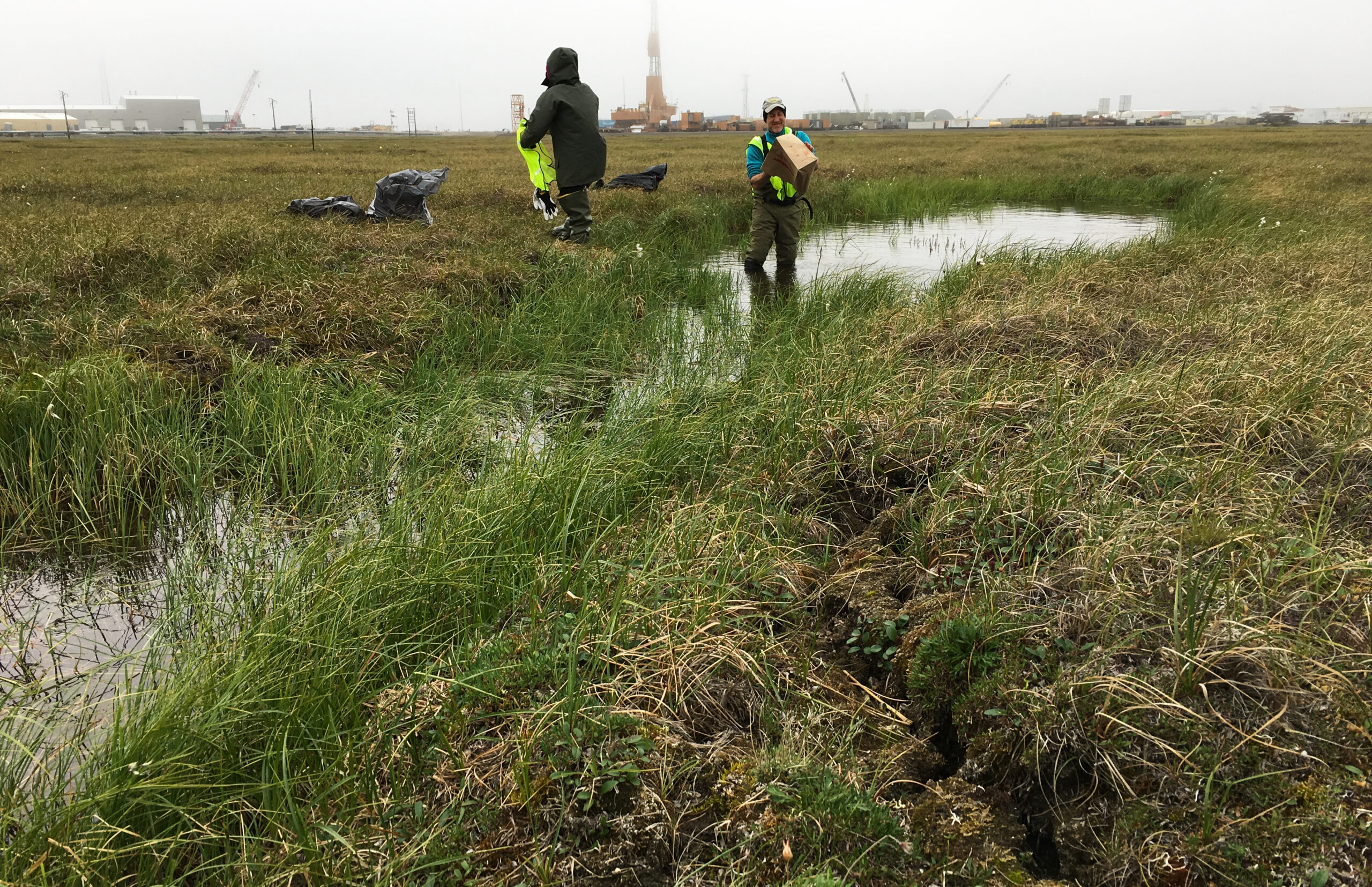
Cracked ground indicates degradation of an underlying ice wedge. A fully frozen wedge pushes the soil above it up to form mounds.
photo by Anna Liljedahl
“Our future goals for the Gateway would utilize new AI models to identify trends or patterns or relationships between ice wedge polygons and elevation, soil or climate data,” says Liljedahl.
How do neural networks work?
The projects above are examples of neural-network-based AI. But how do they actually work?
The comparison to human brains is apt. The networks are composed of interconnected, mathematical components called “neurons.” Also like a brain, the system is a web of billions upon billions of these neurons. Each neuron carries a fragment of information into the next, and the way those neurons are organized determines the kind of tasks the model can be trained to do.
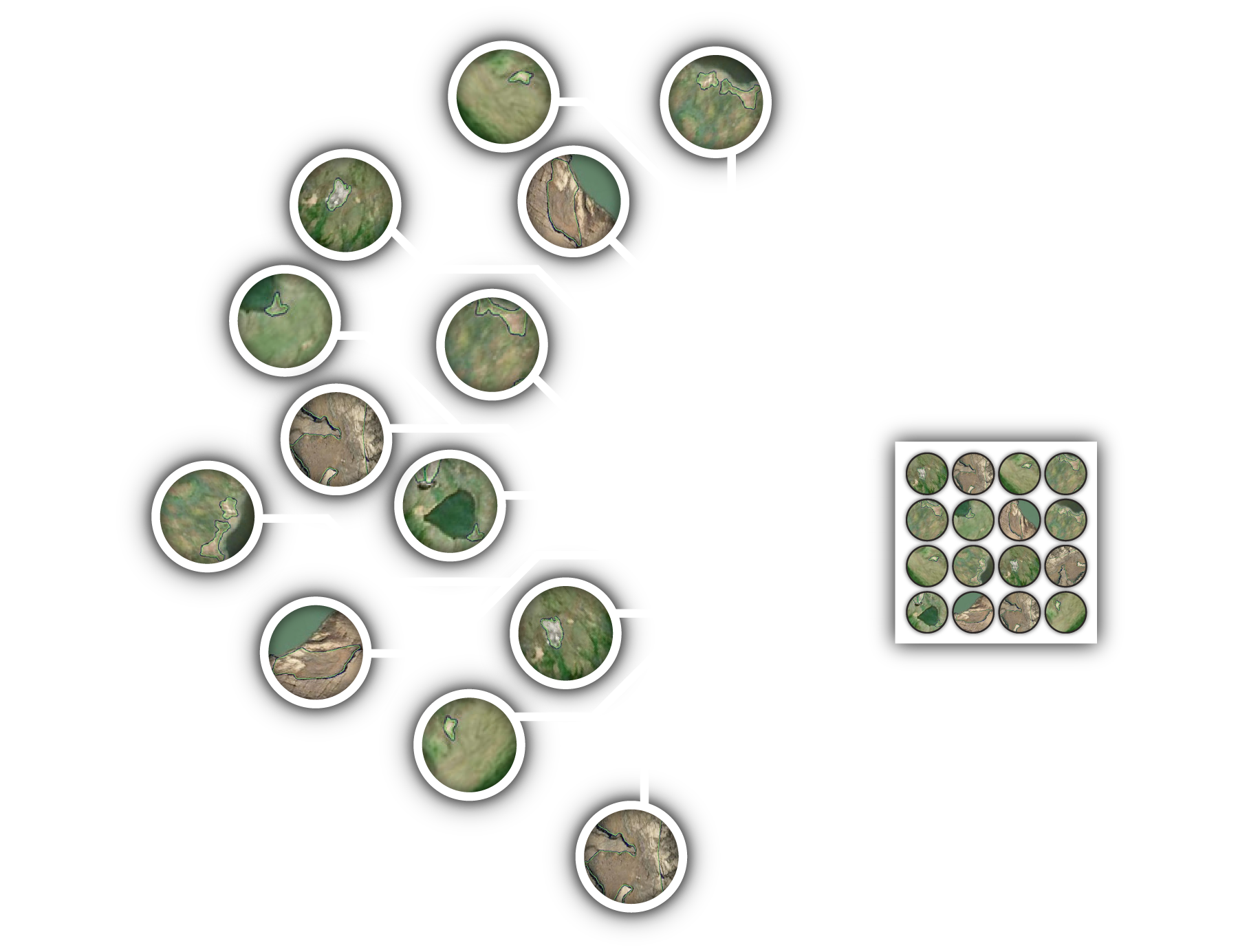
An interconnected web of neurons composes a neural network.
graphic by Julianne Waite
“How AI models are built is based on a really simple structure—but a ton of these really simple structures stacked on top of each other. This makes them complex and highly capable of accomplishing different tasks,” says Mullen.
In order to accomplish these highly specific tasks, the model has to be trained. Training involves feeding the AI input data, and then telling it what the correct output should look like. The process is called supervised learning, and it’s functionally similar to teaching a student by showing it the correct answers to the quiz ahead of time, then testing them, and repeating this cycle over and over until they can reliably ace each test.
In the case of Yang’s work, the model was trained using input satellite images of the Arctic tundra with known retrogressive thaw slump features. The model outputs possible thaw slumps which are then compared to the RTS labels hand-drawn by Research Assistant Tiffany Windholz. It then assesses the similarity between the prediction and the true slump, and automatically adjusts its billions of neurons to improve the similarity. Do this a thousand times and the internal structure of the AI starts to learn what to look for in an image. Sharp change in elevation? Destroyed vegetation and no pond? Right geometry? That’s a potential thaw slump.
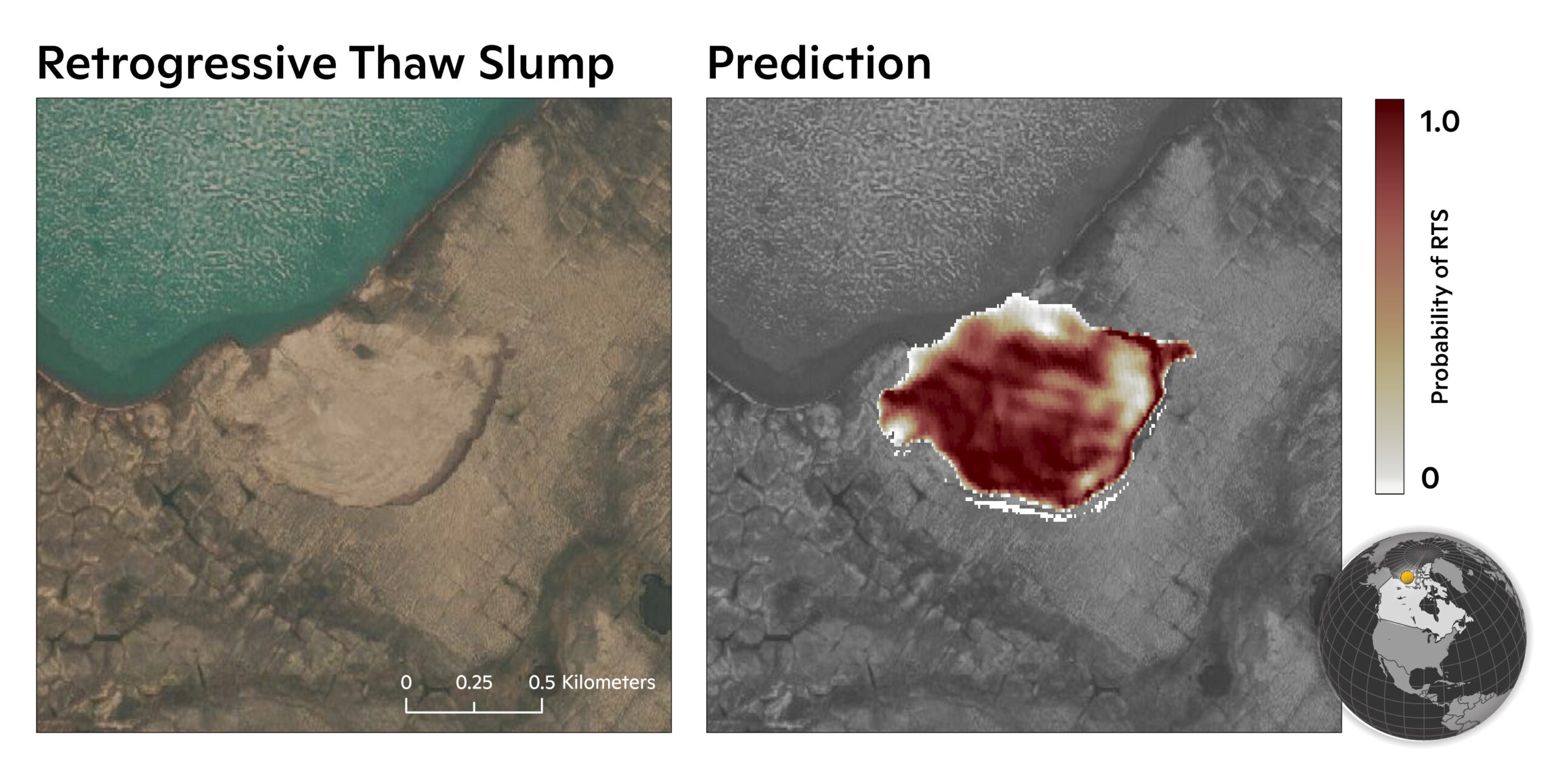
Comparison of satellite imagery with the AI model prediction of where a thaw slump might be.
graphic by Greg Fiske
Just as it would be impossible to pull out any single neuron from a human brain and determine its function, the complexity of a neural network makes the internal workings of AI difficult to detail—Mullen calls it a “black box”—but with a large enough training set you can refine the output without ever having to worry about the internal workings of the machine.
Speeding up and scaling up
Despite its reputation in pop culture, and the uncannily human way these algorithms can learn, AI models are not replacing human researchers. In their present form, neural networks aren’t capable of constructing novel ideas from the information they receive—a defining characteristic of human intelligence. The information that comes out of them is limited by the information they were trained on, in both scope and accuracy.
But once a model is trained with enough accurate data, it can perform in seconds a task that might take a human half an hour. Multiply that across a dataset of 10,000 individual images and it can condense months of image processing into a few hours. And that’s where neural networks become crucial for climate research.
“They’re able to do that tedious, somewhat simple work really fast,” Mullen says. “Which allows us to do more science and focus on the bigger picture.”
Francis adds, “they can also elucidate patterns and connections that humans can’t see by gazing at thousands of maps or images.”
Another superpower of these AI models is their capability for generalization. Train a model to recognize ponds or ice wedges or thaw slumps with enough representative images and you can use it to identify the water bodies across the Arctic—even in places that would be hard to reach for field data collection.
All these qualities dramatically speed up the pace of research, which is critical as the pace of climate change itself accelerates. The faster scientists can analyze and understand changes in our environment, the better we’ll be able to predict, adapt to, and maybe lessen the impacts to come.